Working at the Edge
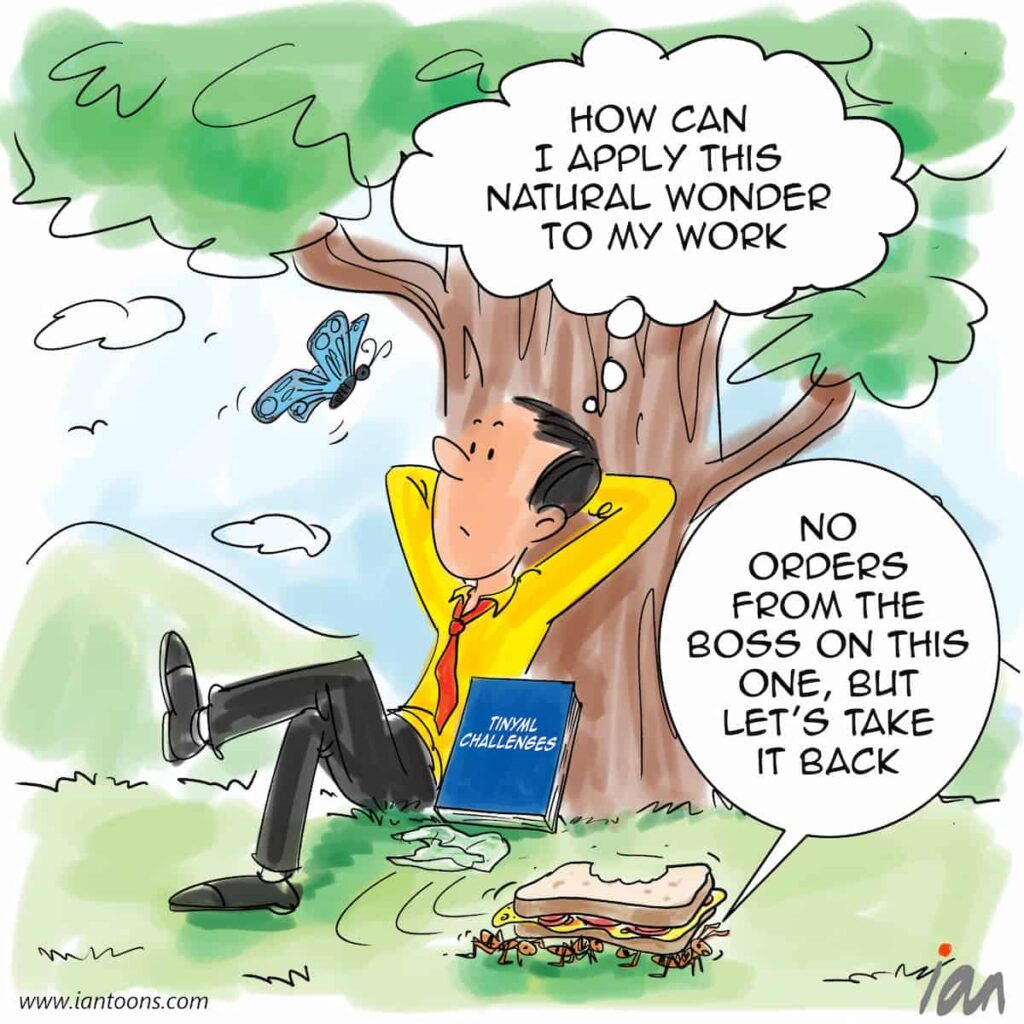
“Working at the Edge” – a cartoon that illustrates how nature can perhaps give a guide on how to improve intelligence at the edge of the computing networks.
The debate about where to place computing intelligence at the center or the edge of a network has gone on for over fifty years. With the advances in machine learning, this debate has transferred to what level of localized control should be allowed by TinyML.
TinyML is a type of machine learning that allows models to run on smaller, less powerful devices, which take up a small footprint.
Example TinyML use cases are predictive maintenance, image traffic optimization, noise monitoring, and other services that can run locally on distributed sensors and cameras without needing to take direction from a central database.
Consumer use cases today are keyword spotting in voice technology solutions, for example when we call “Hey Siri..” a TinyML function with low battery/intelligence usage wakes up the central database.
However, some of the challenges of TinyML is the fragmented hardware environment and how to get the different devices with TinyML to work together.
One area that might prove instructive to this issue is how ants organize, managing to make localized decisions and use antenna touch to communicate with each other.
According to Professor Bernd Meyer, from the University of Monash in Australia, ants “make quite complicated decisions without the help of a logistics expert.”
He added, “the way ants organize themselves can give us insights into things like better traffic flow management and factory floor optimization.”
14
0
0